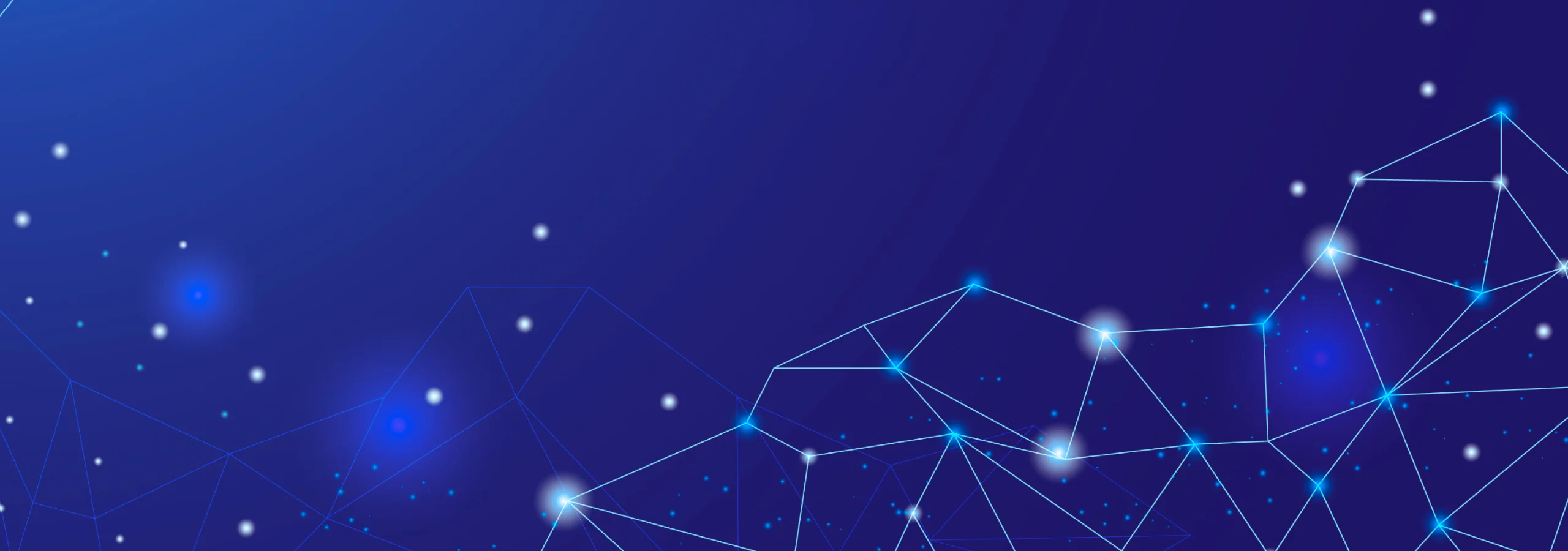
Bits & Bytes: The IT Chronicles
Strategies for Overcoming AI Implementation Challenges
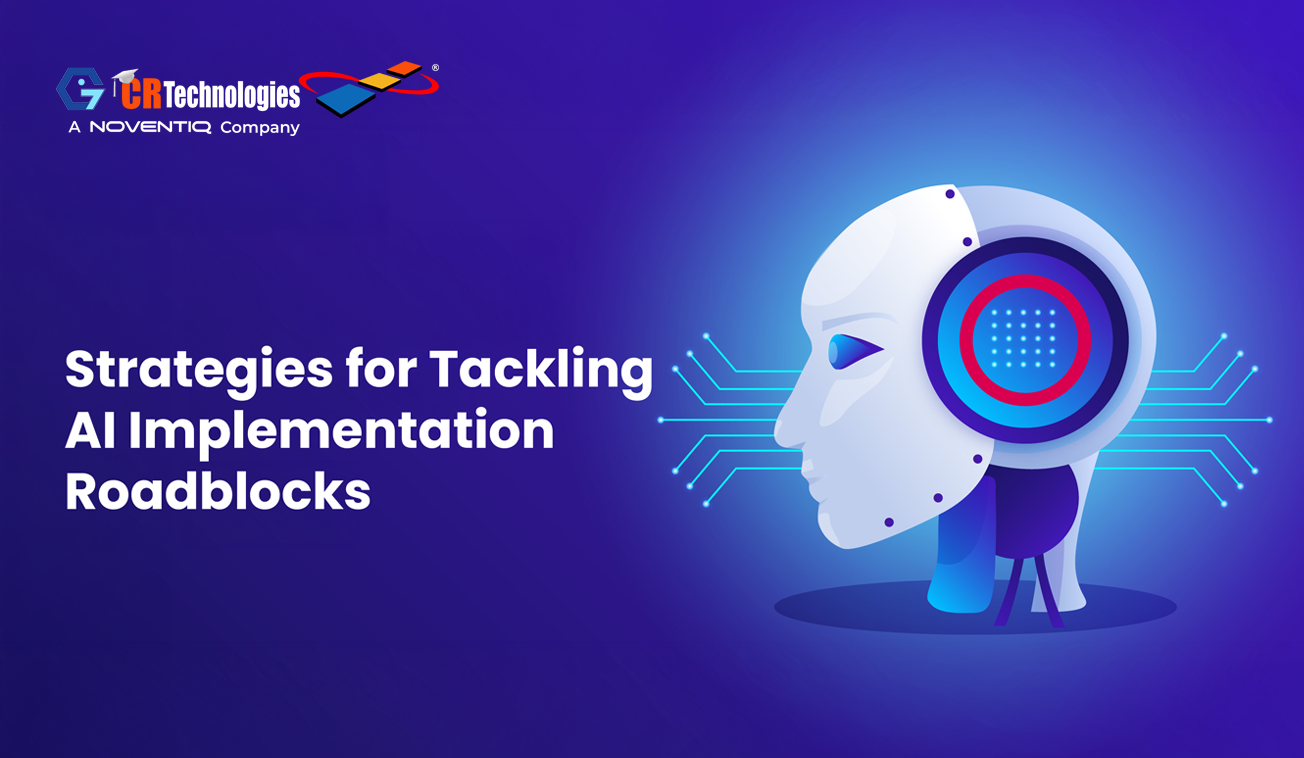
Why Do AI Projects Fail?
AI is now a necessity for businesses looking to improve efficiency, optimize operations, and drive growth. Despite its potential, many AI initiatives fail before they deliver real value.
A 2023 Gartner report found that only 54% of AI projects make it past the pilot stage due to challenges such as poor data quality, lack of internal expertise, integration issues, and resistance to change.
If you are facing AI implementation challenges, you are not alone. This article outlines practical strategies to overcome these roadblocks and ensure AI delivers a measurable business impact.
1. Define Clear Business Objectives Before Implementing AI
One of the biggest reasons AI implementation projects fail is unclear goals. Many companies adopt AI because it’s trending, not because they have a clear problem to solve.
Strategy
Identify specific business challenges AI can address (e.g., reducing operational costs, improving customer engagement, or optimizing supply chains).
Set measurable KPIs such as accuracy improvement, cost reduction, or time saved per task.
Align AI implementation goals with overall business strategy rather than treating it as a standalone tech upgrade.
Example: Instead of just “AI implementation for customer service,” set a goal like “reducing response time by 40% through AI-driven chatbots.”
2. Ensure High-Quality, Well-Structured Data
AI is only as good as the data it is trained on. Poor data quality, fragmented datasets, and biases can lead to inaccurate predictions and unreliable AI performance.
Strategy:
Conduct a data audit to assess data completeness, consistency, and relevance before training AI models.
Use data cleaning and validation processes to remove duplicate, outdated, or biased data.
Implement data governance policies to ensure structured, high-quality data collection across all departments.
A MIT Sloan study found that dirty data costs businesses an average of $12.9 million per year due to errors and inefficiencies.
3. Start Small with Pilot Projects Before Scaling AI
Many AI implementation projects fail because companies try to implement large-scale AI solutions without testing feasibility first. A phased approach minimizes risks and allows for adjustments before full deployment.
Strategy:
Beginning with a small-scale AI pilot focused on a single department or function.
Analyze the results and gather feedback to fine-tune the model before expanding AI across the organization.
Scale AI gradually based on proven success metrics.
Example: Instead of deploying AI across all customer service channels at once, start with an AI chatbot for FAQs and track its effectiveness before expanding to other areas.
4. Address Employee Resistance Through Training and Change Management
AI implementation often fails due to fear of job loss and lack of understanding. Employees may resist AI-driven automation if they see it as a threat rather than a tool for productivity.
Strategy:
Conduct AI awareness sessions to educate employees on how AI enhances rather than replaces human roles.
Provide hands-on training programs to help employees learn to work alongside AI systems.
Encourage a culture of AI adoption by highlighting AI-driven successes within the company.
5. Ensure AI Integrates Seamlessly with Existing IT Infrastructure
AI implementation often faces delays because it does not integrate well with legacy systems, requiring expensive overhauls or complex workarounds.
Strategy:
Choose AI solutions that are compatible with your existing software and workflows.
Implement API-based AI tools that connect with your current CRM, ERP, and analytics platforms.
Work with AI vendors that offer flexible, customizable AI models for easier integration.
Example: AI-driven predictive analytics should pull data from your existing databases and cloud storage and not require a separate data pipeline.
6. Manage AI Costs and Prove ROI
AI projects can be expensive, and unclear ROI can lead to budget cuts or abandonment. Businesses must ensure AI delivers measurable value.
Strategy:
Calculate AI costs vs. expected savings (e.g., reduced labor costs, improved efficiency, revenue growth).
Measure AI success through tangible KPIs like reduced operational time, increased sales conversions, or cost savings.
Take advantage of cost-effective AI solutions such as G7 CR Technologies’ free $10K AI implementation to reduce upfront investment risks.
7. Choose the Right AI Vendor for Long-Term Success
Selecting the wrong AI vendor can lead to security risks, failures, and poor implementation. Businesses should work with partners that understand both AI technology and industry-specific challenges.
Why G7 CR Technologies – A Noventiq Company is the Right AI Partner
✔ Industry-Specific AI Solutions – Tailored AI models for healthcare, finance, retail, and more.
✔ Security & Compliance – AI that meets all the regulations.
✔ Seamless Integration – AI solutions designed to work with your existing infrastructure.
✔ Proven Expertise – Helping businesses scale AI with ethical, bias-free, and cost-effective solutions.
✔ $10K Free AI Implementation – Start your AI journey without upfront costs.
AI implementation comes with challenges, but businesses that approach it strategically can achieve real, measurable success. By setting clear objectives, ensuring data quality, starting with pilot projects, and addressing employee adoption, companies can reduce risks and maximize AI’s potential.